Research
Theory of Cooperation
Cognitive and mathematical principles of cooperation, and their application to the systems in wide range of fields are studied.
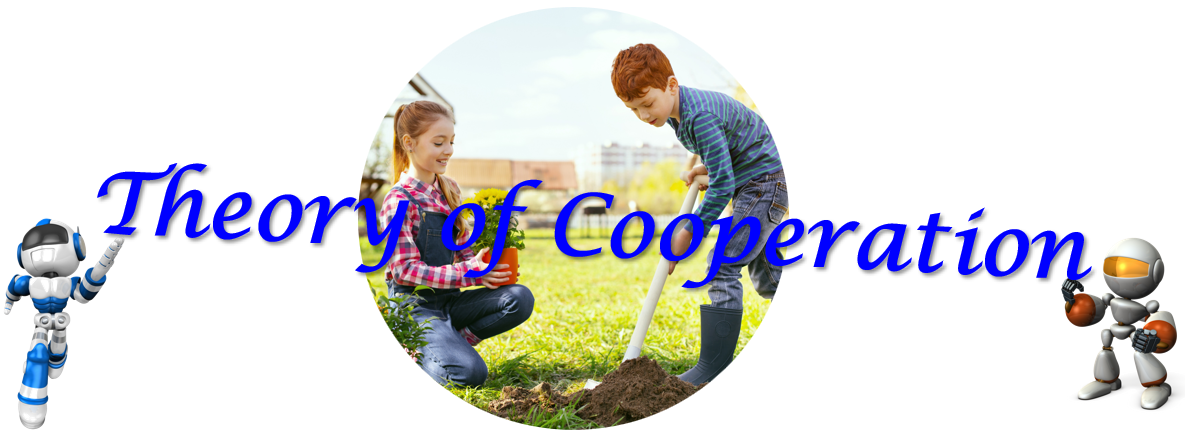
Interactive software "RoCoCo" for theory of cooperation has been developed.
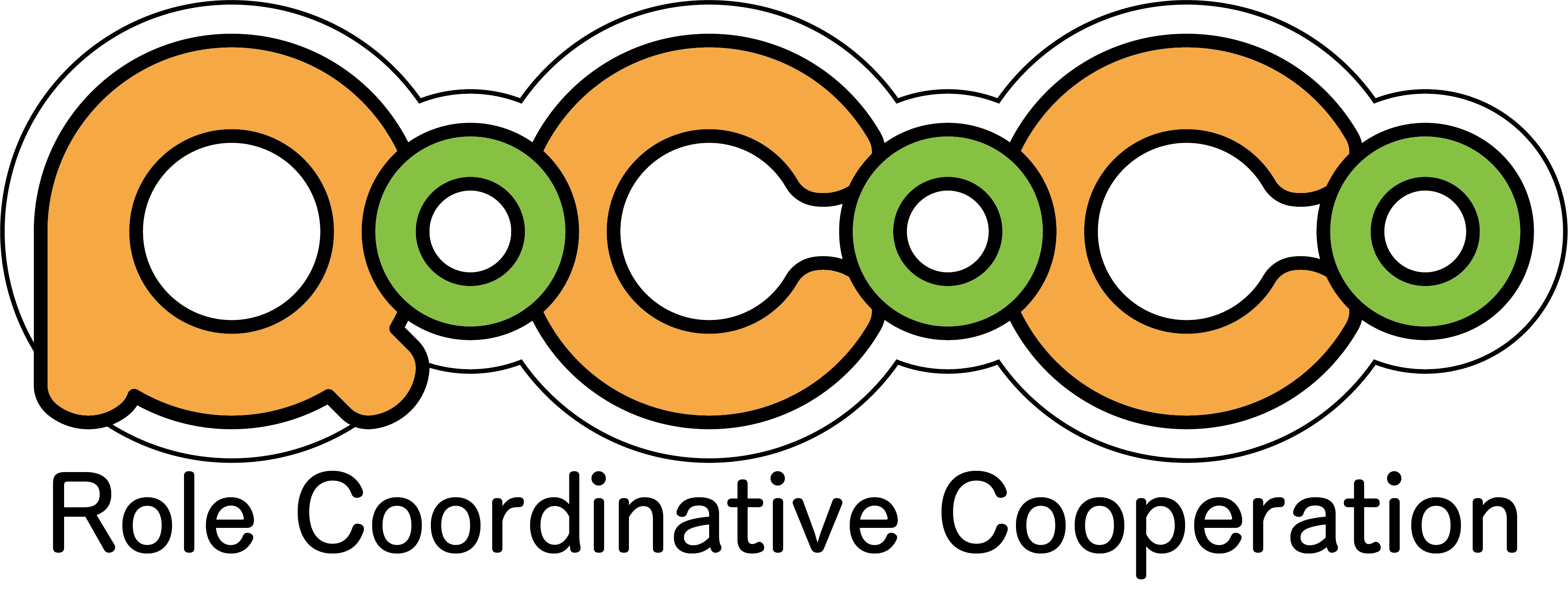
- 岩橋直人 “人工知能” 人間の許容・適応限界事典, IX-05, pp.677-683, 朝倉書店, 2022. PDF
- 岩橋直人 “協力する知能をつくる ―運転から言語獲得までを統べる協力の数理 ” 人工知能を用いた五感・認知機能の可視化とメカニズム解明, 技術情報協会, 2021
- Video for RoboCup@Home Competition 2020. [YouTube]
Physics Projection
A new approach named physics projection enables robots to learn the physical world and predict the effects of their actions actively and online. Physics projection consists of three components: a robot, physical world model, and physics engine. The process of physics projection has a double loop structure comprising (1) a learning loop of the physical world model and (2) a simulation search loop.
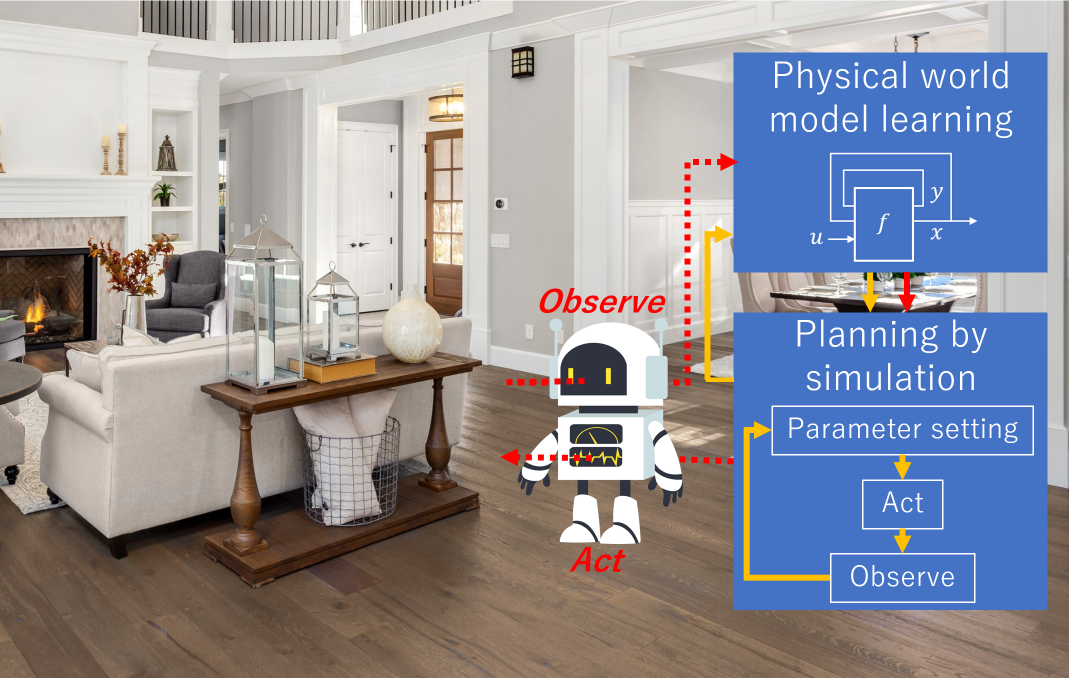
- 【特許登録】岩橋直人, 川野壮一, 新規物体操作ロボットの制御プログラムおよび制御方法、ならびに、新規物体操作システム(Physics Projection), 特許第6921448号, 2021
- Naoto Iwahashi. “Physics Projection,” IEEE Int. Conf. on Awareness Science and Technology, 2019. , 2019. PDF
Developing Intelligence
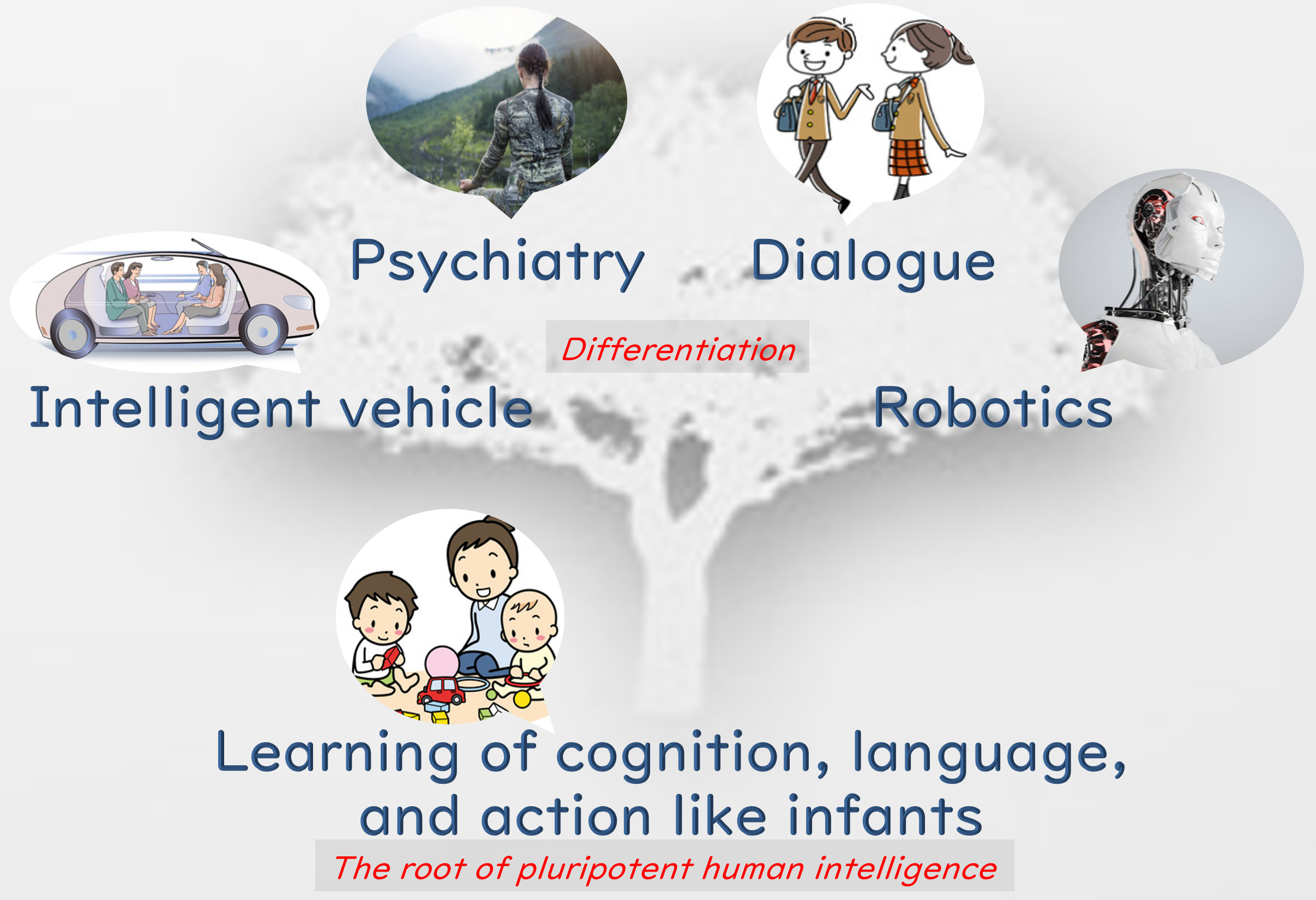
Language Acquisiton Robots
ロボットが人間と経験を共有した日常的な言語コミュニケーションを行えるようになるためには,ロボットが,感覚・運動系などの認知機能との関連性を含めた総体としての言語システムを,人間や環境との相互作用を通して,適応的に学習する仕組みをいかに実現するかが課題です.この課題に対し,当研究室は,従来とはまったく異なるヒューマン・ロボット・インタラクション研究のアプローチ ―発達的アプローチ― の研究を推進しています.本アプローチに基づいて,これまでに,動作と言語によるマルチモーダルコミュニケーション能力を学習する手法を開発してきました.本手法によれば,ロボットは,初期状態において,言語のような記号的な情報を全く持たず,発話や行動による人間との共同行為を通して,言語,物体概念,ならびに動作を,オンラインでインクリメンタルに学習できます.その結果,ロボットは,状況に応じて,人間の発話を解釈し,日用品や縫いぐるみを操作したり,人間に操作指示や確認の発話をしたり,質問に答えたりできるようになります.
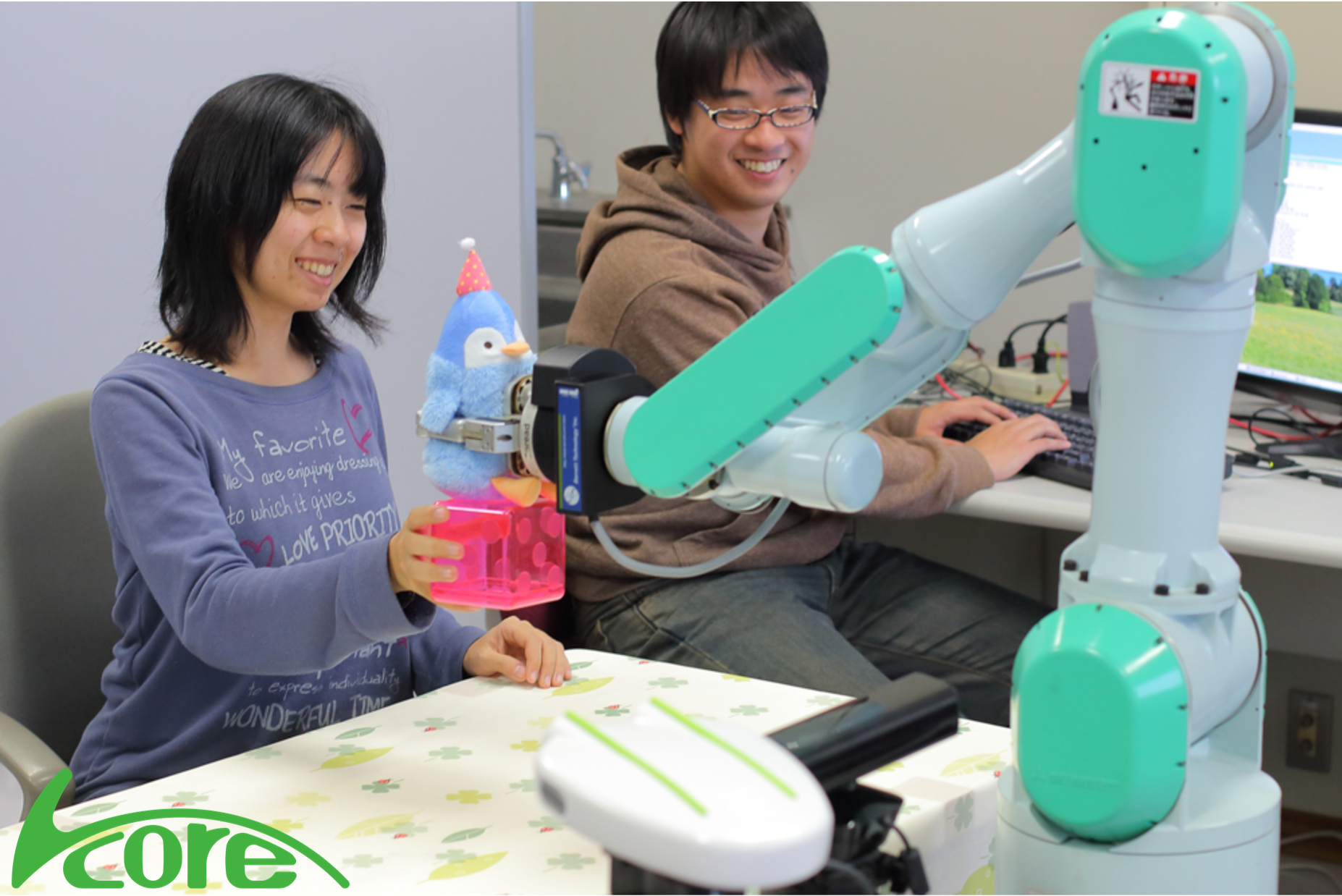
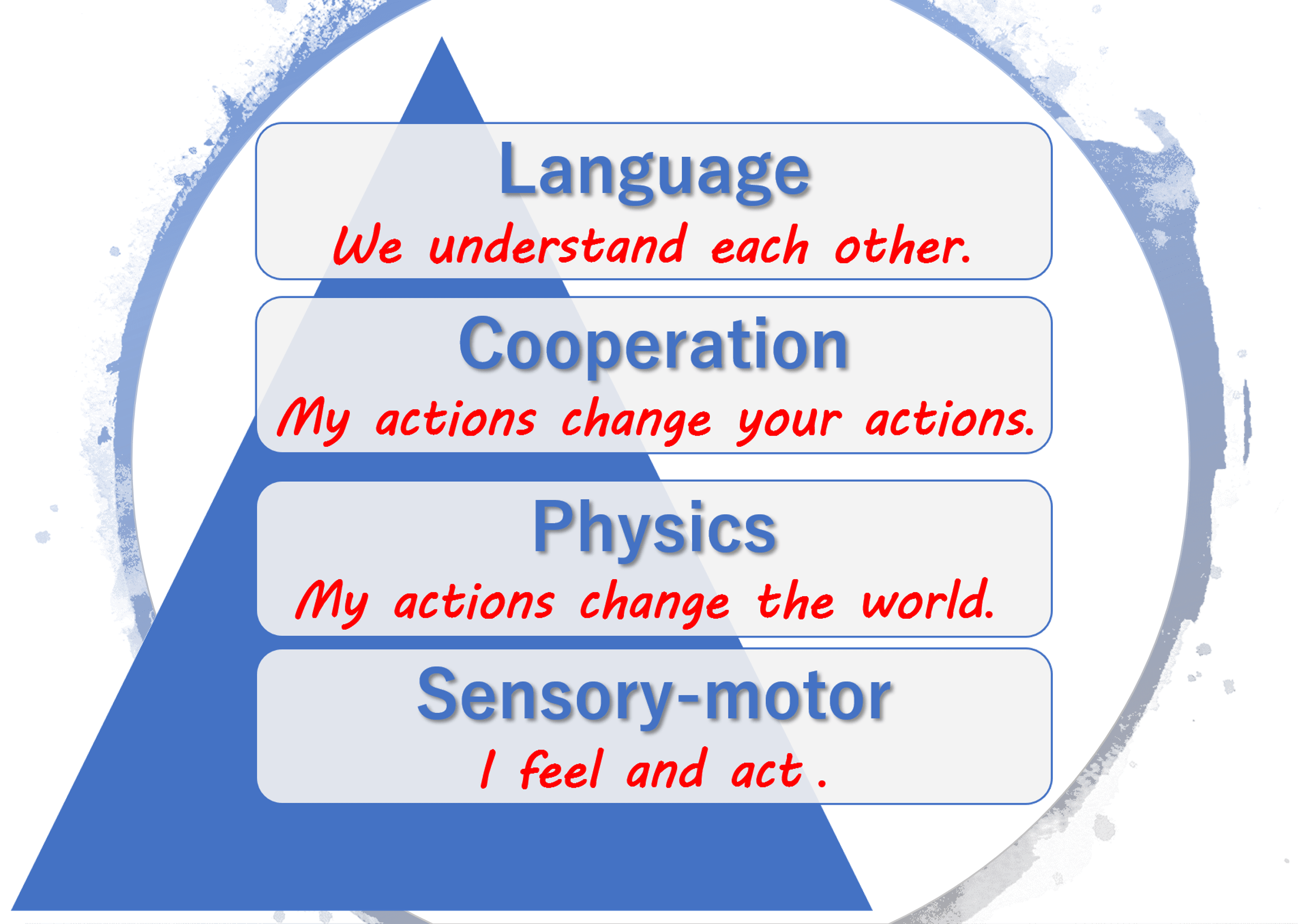
References
- 岩橋直人, “ロボットによる言語獲得 -言語処理の新しいパラダイムを目指して,” 人工知能学会誌, vol.18, no.1, pp.49-58, 2003.PDF
- N. Iwahashi. “Robots That Learn Language: A Developmental Approach to Situated Human-Robot Conversations” in Human-Robot Interaction, N. Sarkar, Ed. Vienna: I-Tech Education and Publishing, 2007, pp.95-118.
- N. Iwahashi, K. Sugiura, R. Taguchi, T. Nagai and T. Taniguchi, “Robots That Learn to Communicate: A Developmental Approach to Personally and Physically Situated Human-Robot Conversations,” in Proc. AAAI Fall Symposium on Dialog with Robots, 2010, pp.38-43.
- 杉浦孔明, 岩橋直人, 柏岡秀紀, 中村哲. “言語獲得ロボットによる発話理解確率の推定に基づく物体操作対話,” 日本ロボット学会誌, vol.28, no.8, pp.978-988, 2010.
Neural Network Model of Human-Robot Multimodal Language Interaction
事前に言語的な知識(単語,文法など)や物体の知識(物体クラスなど)をまったく与えずに、人間の行為(言語・行動)に対して,ロボットがどのような行為(言語・行動)で応答すればよいのかを学習できるディープラーニングの新しい手法を開発した.
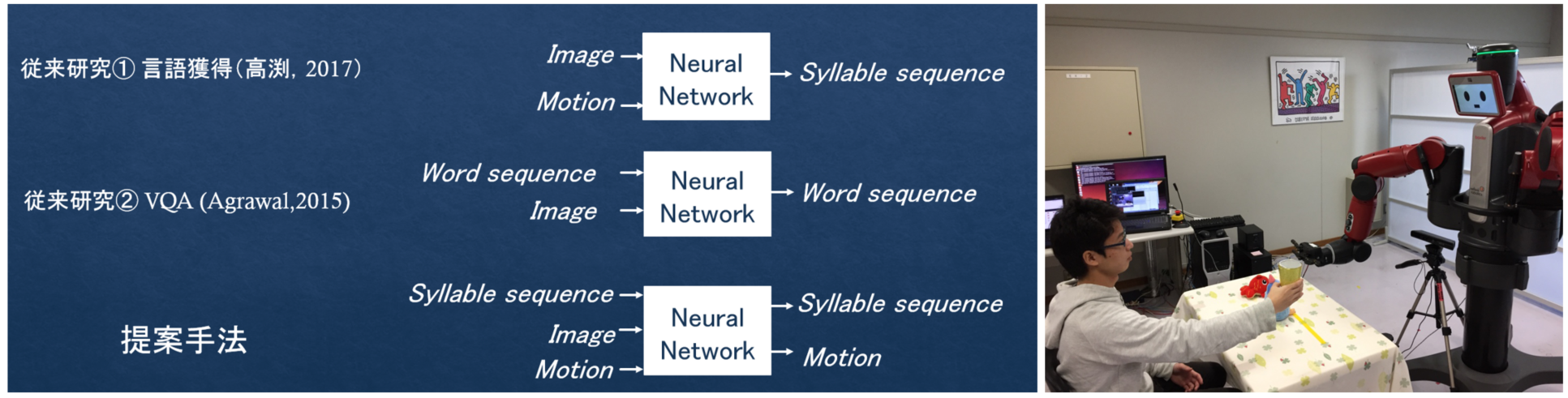
References
- 守屋綾祐,高渕健太,岩橋直人,“Neural Network Model for Human-Robot Multimodal Linguistic Interaction,” ヒューマン・エージェント・インタラクション・シンポジウム 2017.PDF
Recurrent Grad-CAM: Spatiotemporal Localization for Explaining Videos
ディープラーニングに基づき画像・動画を処理して,認識・キャプション生成・質問応答生成・新規画像生成・自動運転などを行う技術の性能が飛躍的に向上し,多くのタスクで人間の能力を超え始めている.こうした人工知能を有効に利用し,豊かな人間社会を作り上げるためには,人工知能と人間が協調しあうメカニズムが必要であり,今まさにそのような技術の開発に注目が集まっている.本研究室では,ディープラーニングが,動画の時空間のどの部分に着目して高度な判断を行ったのかを可視化する技術の開発に成功した.
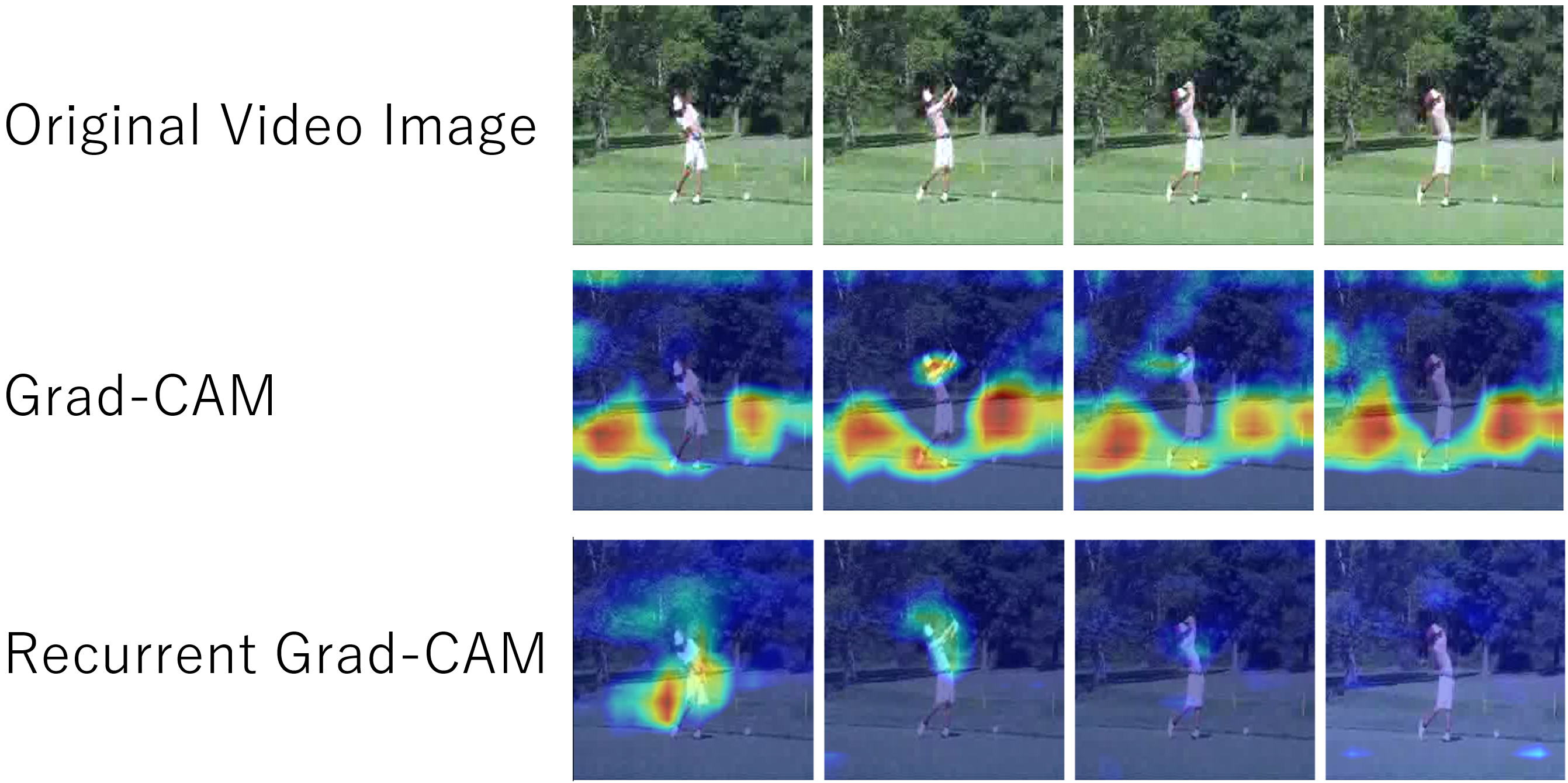
References
- N. Yamashita, N. Iwahashi, S. Nakano, T, Sakai and M. Hamano. “ Visually Explaining Videos using Recurrent Neural Networks with Gradient-Based Localization,” 情報処理学会全国大会, Mar. 2018.PDF
Context Dependent Intentional Motion
下図に示す画像中で,人間によって動かされている縫いぐるみの移動は,画面中央で静止している縫いぐるみを参照点にとれば「飛び越える」という概念の例であり,画面右側の箱を参照点にとれば「乗る」という概念の例になります.このように空間的移動の概念には,参照点に依存しているものがあります.このような参照点に依存する動作を学習・認識・生成するための手法,参照点に依存した隠れマルコフモデル(Referential-Point-Dependent hidden Markov model (RPD-HMM))を提案し,さらに高性能なディープラーニング技術を開発しました.
References
- 羽岡哲郎, 岩橋直人. “言語獲得のための参照点に依存した空間的移動の概念の学習,” 電子情報通信学会技術研究報告 PRMU2000-105, 2000, pp.39-46.PDF
- K. Sugiura, N. Iwahashi, H. Kashioka and S. Nakamura. “Learning, Generation and Recognition of Motions by Reference-Point-Dependent Probabilistic Models,” Advanced Robotics, vol.25, no.6-7, pp.825-848, 2011.
- 深井海生, 武井豪介, 高渕健太, 岩橋直人, Ye Kyaw Thu, 國島丈生. “LRCNによる参照点に依存した動作の認識,” 人工知能学会全国大会, Mar. 2017. PDF